
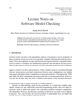
Birthweight (BW) is a critical marker for neonatal health and subsequent development. Neonates born with low BW (LBW) face heightened risks of various health complications, both immediate and long-term. While previous research has predominantly delved into physiological, ultrasound, and lifestyle determinants of BW, recent clinical studies indicate a potential association between neonatal BW and maternal nutrition, including factors like vitamin B12, plasma folate, and iron levels. However, this area remains largely unexplored in terms of predictive analytics. This study aims to bridge this gap by exploiting various machine learning (ML) models to estimate BW and investigate the influence of maternal nutritional factors on BW. Leveraging a cohort of 729 pregnant women monitored during their second trimester in Reus and Tarragona, Spain, the extreme gradient boost model demonstrated robust predictive performance, achieving a mean absolute error (MAE) of 210 grams and an R-squared (R2) value of 0.857. The findings of this study not only validate the predictive significance of maternal nutritional status but also underscore plasma folate and Vitamin B12 as the most influential predictors of BW. Furthermore, the predictive capacity of the proposed model is clarified through partial dependence plots on how variations in maternal factors significantly affect BW.