
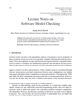
Multiple sclerosis (MS) is a chronic autoimmune disease that predominantly affects the central nervous system (CNS) and is a leading cause of neurological disability among young adults. MS diagnosis heavily relies on clinical symptoms coupled with the detection of demyelinating lesions in the CNS, as depicted in conventional magnetic resonance images (MRI). These lesions may evolve over time and serve as critical biomarkers for assessing disease activity and the effectiveness of therapeutic interventions. Manual lesion delimitation in images is a tedious and error-prone process, prompting active research into automated systems. MS lesion segmentation can be conducted in two very different approaches: cross-sectionally: by using a single MRI, or longitudinally, by identifying changes such as new lesions or variations in lesion size across consecutives MRIs. Deep learning architectures such as U-Net, which employs convolutional neural networks with skip connections, have proven effective for MRI lesion segmentation. In this paper, we utilize the nnUNet v2 architecture and introduce an enhanced method capable of segmenting both cross-sectional and longitudinal MRIs. We used the ISBI 2015 dataset, training our U-Net model on a subset of patients using a generative pipeline and testing on another subset of patients from the same dataset. For training the model, we used a Bayesian generative approach to create different synthetic data simulating random variations in lesion masks. In this process, we have randomly simulated multiple temporal variations of the lesion masks, including erosion, dilation, and the removal of individual lesions within each mask. Then, we adapted the U-Net to train using a single MRI and its corresponding synthetic segmentation, which simulates the lesions in a previous time point. Thanks to the large amount of synthetic data generated, the U-Net is able to learn the intrinsic behavior of the lesions which include expansion, shrinkage, appearance and disappearance. This approach enables, for the first time, the two classical operational modes for MS lesion segmentation within a single model: cross-sectional segmentation, where the model receives a MRI and an empty mask as input, and longitudinal segmentation, where the input includes a MRI and a mask from a previous time point. Our approach achieved a Dice Similarity Coefficient (DSC) of 0.75 for cross-sectional segmentation and 0.81 for longitudinal segmentation, indicating improved performance when incorporating temporal information.This study demonstrates that it is possible to apply a generative method in conjunction with a U-Net architecture for both cross-sectional and longitudinal MRI lesion segmentation in MS, yielding promising results.