
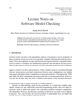
In an era characterized by the rapid evolution of data-driven applications, the generation of high-quality synthetic data has become increasingly indispensable. This serves as a crucial element for advancing research, development, and ensuring the responsible management of sensitive information. However, the synthesis of fundus images presents unique challenges due to the intricate and highly detailed structures inherent in retinal images. While Generative Adversarial Networks (GANs) show promise in image synthesis, they often encounter training difficulties and struggle to produce truly realistic images. This paper introduces SynthRetina, an innovative system that harnesses the capabilities of GANs to generate lifelike fundus images. SynthRetina amalgamates a generator network and a discriminator network, facilitating the creation of synthetic fundus images with diverse applications across the medical field. The generator network specializes in transforming input fundus images from one class to another, while the discriminator network rigorously evaluates the authenticity of the generated images. SynthRetina effectively addresses the challenge of limited availability of medical data for research and development, offering a solution that enhances data augmentation and improves the performance of fundus image classification tasks. An evaluation of the SynthRetina architecture using a real fundus image dataset demonstrates its ability to produce a more diverse and realistic collection of fundus images compared to other GAN-based methods.