
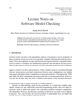
The future faces escalating water scarcity due to population growth, climate change, and inefficient resource management. Therefore, innovative solutions for sustainable access and usage are needed. Seawater Reverse Osmosis (SWRO) desalination stands out as a key technology in tackling this dilemma. However, SWRO is energy-intensive, primarily due to the need to pressurize seawater to overcome the osmotic pressure to produce fresh water. In this regard, real-time management of operating parameters in SWRO plants enables minimizing energy consumption and chemical usage and adjusting water production in response to demand and water conditions, highlighting the need for real-time monitoring and advanced simulation tools such as digital twins. In response, this study explores the potential of eleven machine learning algorithms to simulate the SWRO process using a vast dataset of 18.816 scenarios generated through a solution diffusion transport model. Our investigation covers both non-ensemble and ensemble models. Additionally, a Shapley additive explanation analysis was carried out to gain insights into the most influential predictors and confirm the model’s ability to comprehend the Reverse Osmosis (RO) process. The findings underscore the high accuracy of the algorithms, particularly XGBoost, CatBoost and ANN, in predicting key parameters such as permeate flow, permeate salinity and specific energy consumption. Furthermore, Support Vector Machine regression model shows promising in predicting permeate flow. These findings highlight the potential of data-driven models, particularly ensemble-based algorithms, in simulating SWRO behavior, laying the groundwork for future process optimization.