
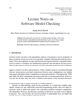
This study delves into the realm of healthcare data analysis within the framework of local differential privacy (LDP), aiming to assess the performance of various methodologies under this stringent privacy paradigm. Leveraging LDP, which ensures individual data privacy while allowing for analysis, we explore the landscape of healthcare data with a focus on meaningful performance evaluation. Through a systematic investigation, this research evaluates the performance and efficiency of different approaches, shedding light on their suitability for healthcare data applications. By synthesizing insights from diverse techniques and considering their implications within the context of LDP, this study contributes to the advancement of privacy-preserving healthcare analytics while maintaining the integrity and utility of the underlying data. Our findings indicate that Reduced and Castell approaches present the best performance while maintaining a low epsilon, ε < 1. The Castell approach, with its lower memory consumption than the Reduced approach, stands out as the best approach for large healthcare datasets.