
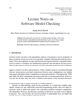
Video Anomaly Detection (VAD) is a well-established area of research with significant potential for enhancing video surveillance in urban public transportation. However, current VAD systems often propose powerful methodologies but overlook their use in extreme environments like public transportation, necessitating a balance between performance and computational efficiency. In this paper, we evaluate a key component in many VAD frameworks: feature extractors. We investigate five extractors: Inflated 3D ConvNets (I3D), 3D Convolutional Neural Networks (C3D), Unified Transformer (UniFormer) in Small (UniFormer-S) and Base (UniFormer-B) versions, and Temporal Shift Module (TSM). These are integrated into a VAD architecture employing Bidirectional Encoder Representations from Transformers (BERT) with Multiple Instance Learning (MIL), chosen for its modularity and clear separation between the feature extractor and anomaly detector module. UniFormer-S demonstrated a processing rate of 4.64 clips per second with a computational demand of 28.717 GFLOPs on edge devices like the Jetson Orin NX (8GB RAM, 20W power). On the UCF-Crime dataset, UniFormer-S with BERT + MIL achieves an AUC of 79.74%. These findings highlight the promise of UniFormer-S and the use of edge devices like the Jetson Orin NX in public transportation due to their balance of performance and efficiency.