
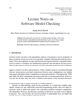
This study investigates the application of machine learning to enhance the accuracy of saturated flow boiling heat transfer correlations. Traditional correlations often exhibit limitations, motivating the exploration of alternative approaches. A comprehensive dataset encompassing 2770 experimental observations, compiled from over 15 published research papers, serves as the foundation for this research. The data incorporates flow boiling heat transfer for pure and mixture refrigerants in smooth tubes. The study compares three machine learning models including neural network, linear regression, and SVM. The data is strategically divided: 80% for training the models and 20% for testing. The wide neural network shows that the best-performing model achieves a Root Mean Square Error (RMSE) of 0.202, demonstrating exceptional prediction accuracy, followed by the linear regression, and SVM training models. Heat Transfer Coefficient (HTC) is predicted with an error of less than 5% for all refrigerants, further confirmed by a high coefficient of determination (0.99). This signifies a significant improvement in traditional experimental-based correlations. Furthermore, the study demonstrates the efficacy of machine learning models in predicting heat transfer enhancement methods. In conclusion, this work highlights the potential of machine learning for refining saturated flow boiling heat transfer predictions.