
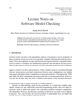
In the realm of forensic science, precise identification of individuals holds paramount importance in both investigative procedures and legal proceedings. Hands and palms recognition has emerged as a valuable biometric modality within forensic applications, owing to the distinct and intricate features inherent to these anatomical regions. The elaborate patterns of veins, creases, and ridges present on palms and fingers serve as rich sources of biometric data, crucial for accurate identification purposes. Furthermore, given the frequent involvement of hands and palms in criminal activities such as theft and assault, their recognition becomes imperative for establishing links between suspects and crime scenes. However, developing robust recognition systems tailored for forensic applications poses notable challenges, including variations in hand poses, lighting conditions, and image quality. To address these hurdles, sophisticated deep learning techniques, notably transfer learning, have been employed. By harnessing pre-trained deep learning models namely NasNetLarge, NasNetMobile, and EfficientNet, initially trained on expansive datasets for general image recognition tasks, we can adapt these models to the specific task of hands and palms recognition in forensic contexts. Our findings reveal that all three models consistently achieved over 92% accuracy across all metrics evaluated, demonstrating their efficacy as strong contenders for the hands-and-palms recognition task. Notably, the EfficientNet model exhibited superior performance compared to its counterparts, boasting more than 95.8% accuracy, precision, F1-score and recall, along with more than 98.6% specificity and 99.4% AUC.