
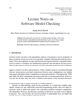
Cyberbullying, marked by its persistent and intentional aggression online, yields severe repercussions for its victims, extending beyond immediate distress to long-lasting effects such as heightened anxiety, depression, and social withdrawal. Individuals subjected to Cyberbullying often grapple with diminished self-esteem, compromised academic performance, and strained interpersonal relations. Given the escalating prevalence of this digital menace, there is a pressing need for advanced methodologies to address it effectively. This paper introduces an approach to Cyberbullying detection, integrating techniques such as Bag-of-Words (BoW) and Term Frequency-Inverse Document Frequency (TF-IDF) analyses, along with the parallel processing capabilities of Convolutional Neural Networks (CNNs) and the contextual comprehension provided by Bidirectional Long Short-Term Memory (BiLSTM) networks. Through an experimentation on the latest Ejaz-Choudhury-Razi Cyberbullying dataset, our framework exhibits satisfactory performance in identifying instances of online hostility. These results underscore the potential of our approach to significantly contribute to ongoing efforts aimed at combating Cyberbullying in digital environments.