
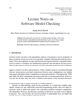
The rapid advancement in deepfake technology has enabled the creation of highly realistic fake images and videos, posing significant risks, especially in the context of explicit content. Such content, which often involves the alteration of an individual’s identity in sexually explicit material, can lead to defamation, harassment, and blackmail. This paper focuses on the detection of deepfakes in explicit content using a state-of-the-art ID-unaware Binary Classification method. We evaluate its effectiveness in real-world scenarios by analyzing three versions of the model with different backbones: ResNet34, EfficientNet-B3, and EfficientNet-B4. To facilitate this evaluation, we curated a dataset of 200 videos, consisting of 100 genuine videos and their corresponding deepfake counterparts, ensuring a direct comparison between genuine and altered content. Our analysis revealed a significant decrease in detection performance when applying the state-of-the-art method to explicit content. Specifically, the AUC score dropped from 93% on standard datasets such as FaceForensics++ to 62% on our explicit content dataset. Additionally, the accuracy for detecting deepfakes plummeted to around 25%, while the accuracy for genuine videos remained high at approximately 90%. We identified specific factors contributing to this decline, including unconventional makeup, lighting issues, and facial blurring due to camera distance. These findings underscore the challenges and the necessity for robust detection methods to address the unique problems posed by explicit content deepfakes, ultimately aiming to protect individuals from the potential harms associated with this technology.