
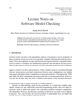
Product reviews represent a valuable source of information for both (potential) customers and sellers. Usually, reviews come in pairs (score, motivation), where the motivation is a piece of unstructured text explaining the score given to a product. For reviews, this setting is ideal to combine a quantitative assessment of a product with a qualitative explanation. Aggregating the numerical scores might be uninformative while parsing large quantities of text might be challenging.
Automated argument analysis can help in this process, and we previously developed an argument-based quality analysis pipeline that helps identify the most significant items from a corpus of reviews. Given that the pipeline is effective but time-consuming, this work sets out to improve its computational efficiency. Next to optimisation by conventional methods, we investigate the effect of reducing the number of text chunks that are used to build the argumentation graph.
We find that conventional methods significantly improve the computation time, which allows us to analyse much larger datasets of real-world reviews. When the number of tokens is scaled down, accuracy remains similar compared to the original version of the pipeline. However, we find that this does not necessarily result in a computation time reduction.