
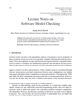
Machine learning (ML) is becoming increasingly important in healthcare decision-making, requiring highly interpretable insights from predictive models. Although integrating ML models with knowledge graphs (KGs) holds promise, conveying model outcomes to domain experts remains challenging, hindering usability despite accuracy. We propose semantically describing predictive model insights to overcome communication barriers. Our pipeline predicts lung cancer relapse likelihood, providing oncologists with patient-centric explanations based on input characteristics. Consequently, domain experts gain insights into both the characteristics of classified lung cancer patients and their relevant population. These insights, along with model decisions, are semantically described in natural language to enhance understanding, particularly for interpretable models like LIME and SHAP. Our approach, SemDesLC, documents ML model pipelines into KGs, and fulfills the needs of three types of users: KG builders, analysts, and consumers. Experts’ opinions indicate that semantic descriptions are effective for elucidating relapse determinants. SemDesLC is openly accessible on GitHub, promoting transparency and collaboration in leveraging ML for healthcare decision support.