
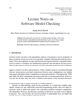
The increasing demand for automatic high-level image understanding, including the detection of abstract concepts (AC) in images, presents a complex challenge both technically and ethically. This demand highlights the need for innovative and more interpretable approaches, that reconcile traditional deep vision methods with the situated, nuanced knowledge that humans use to interpret images at such high semantic levels. To bridge the gap between the deep vision and situated perceptual paradigms, this study aims to leverage situated perceptual knowledge of cultural images to enhance performance and interpretability in AC image classification. We automatically extract perceptual semantic units from images, which we then model and integrate into the ARTstract Knowledge Graph (AKG). This resource captures situated perceptual semantics gleaned from over 14,000 cultural images labeled with ACs. Additionally, we enhance the AKG with high-level linguistic frames. To facilitate downstream tasks such as AC-based image classification, we compute Knowledge Graph Embeddings (KGE). We experiment with relative representations [1] and hybrid approaches that fuse these embeddings with visual transformer embeddings. Finally, for interpretability, we conduct posthoc qualitative analyses by examining model similarities with training instances. The adoption of the relative representation method significantly bolsters KGE-based AC image classification, while our hybrid methods outperform state-of-the-art approaches. The posthoc interpretability analyses reveal the visual transformer’s proficiency in capturing pixel-level visual attributes, contrasting with our method’s efficacy in representing more abstract and semantic scene elements. Our results demonstrate the synergy and complementarity between KGE embeddings’ situated perceptual knowledge and deep visual model’s sensory-perceptual understanding for AC image classification. This work suggests a strong potential of neurosymbolic methods for knowledge integration and robust image representation for use in downstream intricate visual comprehension tasks. All the materials and code are available at https://github.com/delfimpandiani/Stitching-Gaps