
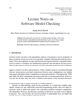
Super-resolution tasks have been receiving widespread attention, contemporary research in the field of single-image super-resolution has seen a notable enhancement in performance, largely attributed to the integration of self-attention mechanisms. However, the disadvantage of the self-attention mechanism is that it significantly increases the amount of computation, which is difficult to accept in lightweight image super-resolution tasks. In this paper, a lightweight image Super Resolution model, Large Kernel Super Resolution (LKSR), is proposed. LKSR simply uses the neural network layer based on large convolutional kernel as the backbone layer. Experimental results show that the proposed LKSR algorithm is effective and offers a promising solution for applications where computational resources are constrained. The LKSR model, with its large convolutional kernels, is designed to capture more contextual information within an image, which is essential for enhancing the resolution without compromising the computational efficiency. This approach allows the model to achieve high-quality image upscaling while maintaining a balance between performance and computational demands, making it suitable for a variety of applications that require both sharp image details and efficient processing.