
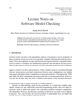
Disease image analysis is vital in various real-world applications, particularly in the field of smart agriculture, where it plays a crucial role in crop disease diagnosis. Given the uncertainties associated with environmental conditions, there is a pressing need to improve the visual quality of crop images. In this paper, we propose an algorithm based on an improved FPAGAN algorithm to address the problem of color distortion and fuzzy texture details of tea disease images caused by low-light environments. Firstly, we combine the feature pyramid module, the attention module and the multi-scale aggregation module to improve the generative network, which effectively reduces the noise effect while increasing the attention to the feature information of the disease region. In order to distinguish between the generated image and the real image, the PatchGAN in the discriminative network is improved and the local discriminative strategy is adopted. Then, the Nash equilibrium is achieved through the continuous interaction between the generator and the discriminator. To guide the network training process, a joint loss function is introduced to suppress the image noise and make the enhanced image color more natural and balanced. Finally, ablation experiments are conducted on the FPANet architecture to verify the advantages of the proposed FPANet. The results show that the FPAGAN algorithm can reach an average value of 19.065, 0.735, and 7.435 on the three evaluation indexes of PSNR, SSIM, and information entropy, and the processed disease images outperform other image enhancement techniques in terms of color preservation, contrast improvement, and enhancement of image details. This holds a certain significance in the prompt detection of tea diseases and minimizing growth expenses.