
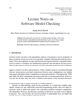
At present, the global urban traffic system generally faces multiple challenges such as congestion problems and environmental loads. Reasonable traffic planning by predicting traffic flow is conducive to solving such problems. As for traffic flow forecasting, we need to establish a forecasting model first. The big data technology is used to process the previous historical traffic data as input, and the traffic data is found through the prediction model to predict the future traffic. The prediction of future traffic is used as the output of the model. In this paper, a new spatio-temporal fusion traffic prediction model based on input traffic signal decomposition is proposed, which is called Decomposed Spatial-Temporal Graph Convolutional Network (DSTGCN), in order to better capture global spatial correlation and time dependence. It captures the global spatial information through the diffusion graph convolution network in the spatial module, and then captures the time information through the designed time module. The spatial module and the time module are organically connected by the forgetting gate and the update gate to decompose and predict the input information in multi-level and two dimensions, and capture temporal and spatial correlation of traffic. Moreover, the residual learning framework is used to enhance the model simulation ability. Experiments on PEMS04 and PEMS08 data sets indicate that the proposed method is more excellent than ASTGCN and Graph WaveNet.