
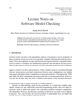
Smart transportation is a new development model for intelligent traffic based on the combination of the Internet, the Internet of Things, and other networks. Road detection is one of the foundational and core technologies in smart transportation. It provides accurate, reliable, and real-time road information for applications such as autonomous driving, intelligent traffic management, and urban planning. This contributes to the improvement of traffic safety, efficiency, and sustainability. However, relying solely on visual images for road detection still faces numerous challenges, such as changes in lighting conditions, image blurring, and occlusions. To enhance the robustness and accuracy of road detection, leveraging LiDAR (Light Detection and Ranging) data as supplementary information is considered. LiDAR data is not affected by visual noise and can offer more precise depth and height information. Nevertheless, effectively integrating and adapting to different data and feature spaces remain crucial challenges. This paper proposes an improved road detection method based on the PLARD (Progressive LiDAR Adaptation for Road Detection) network. It introduces the U-Net network as an additional branch, collaborating with the image and point cloud branches for feature fusion and road detection. The U-Net network utilizes information from the images for initial segmentation, providing a better prior, and enhances the feature fusion capability of the PLARD network, thereby improving segmentation accuracy and robustness. Experiments conducted on the KITTI Road Dataset demonstrate that the proposed method outperforms the PLARD network and other baseline methods in terms of the Dice coefficient. Particularly, it excels in complex urban scenarios and exhibits strong generalization capabilities. The introduced road detection approach, combining LiDAR and visual data, provides an efficient, robust, and versatile solution for smart transportation. It adapts to different scenarios and environments, thereby enhancing the precision and stability of road detection in smart transportation systems.