
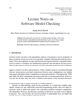
The driver monitoring system constitutes an essential element in the realm of human-machine interaction within intelligent vehicles, primarily tasked with overseeing and promptly alerting deviations from standard driving behaviors that could potentially lead to traffic accidents. Presently, the evolution of driver monitoring systems in China is at a nascent stage. Challenges persist due to constraints in hardware equipment, resulting in relatively simplistic terminal devices for detecting driver fatigue features. Consequently, this has led to frequent occurrences of false positives and missed detections. This study centers around a non-contact vehicle monitoring device employing machine vision. It delves into the development of a rational, effective, real-time, and accurate mechanism for continuously monitoring driving duration while concurrently recording driving behavior data. Within this framework, the research focuses on the utilization of a modified version of MTCNN for real-time driver face detection. It involves extracting critical driver head posture features, encompassing the pitch angle, yaw angle, and roll angle of the driver’s head. By comparing the positions of obtained driver face images, a fatigue driving feature index system is established. This system facilitates the identification, analysis, and discrimination of a driver’s fatigue state based on the extracted head feature values. The ultimate aim is to realize a fatigue warning function within the driver monitoring system, thereby enhancing the detection speed of facial fatigue recognition. This endeavor holds paramount significance for road traffic safety, contributing to the continual improvement of driver monitoring systems and consequently mitigating potential risks on the road.