
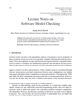
Large language models have gained extensive research interest in the past few years. They have demonstrated remarkable ability to process and generate human-like text, and have improved performances on various natural language processing tasks. This paper is focused on the prompting techniques and knowledge augmentation techniques for text style transfer tasks. Text style transfer involves the transformation of a given sentence in a stylistically different manner while preserving its original meaning. It requires models to understand and manipulate different aspects such as politeness, formality, and sentiment. This paper provides an overview of several methods for prompting large language models for text style transfer and presents an overview of several methods for knowledge augmentation with a discussion about potential use for text style transfer. Preliminary results on formality transfer using the T5 model are presented to evaluate prompting and knowledge augmentation techniques. The results show that using knowledge augmentation techniques improves the performance compared to models without augmentation, while zero-shot prompting techniques are less effective. This emphasizes the necessity of fine-tuning and incorporating knowledge augmentation for enhanced model performance.