
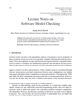
Companies must enhance total maintenance effectiveness to stay competitive, focusing on both digitalization and basic maintenance procedures. Digitalization offers technologies for data-driven decision-making, but many maintenance decisions still lack a factual basis. Prioritizing efficiency and effectiveness require analyzing equipment history, facilitated by using Computerized Maintenance Management Systems (CMMS). However, CMMS data often contains unstructured free-text, leading to manual analysis, which is resource-intensive and reactive, focusing on short time periods and specific equipment. Two approaches are available to solve the issue: minimizing free-text entries or using advanced methods for processing them. Free-text allows detailed descriptions but may lack completeness, while structured reporting aids automated analysis but may limit fault description richness. As knowledge and experience are vital assets for companies this research uses a hybrid approach by combining Natural Language Processing with domain specific ontology and Large Language Models to extract information from free-text entries, enabling the possibility of real-time analysis e.g., identifying recurring failure and knowledge sharing across global sites.