
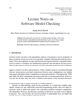
Early diagnosis of chronic heart failure (CHF) is an urgent task in modern medicine. Previously, the authors presented the results of early diagnosis of CHF using neural network classification of static characteristics of plasma and serum. This article aims to solve the problem of classifying the dynamic characteristics of a tensiometric study of the liquid part (plasma and serum) of blood by classifying multidimensional time series. For training the neural network, a database of results of tensiometric studies of the liquid part of the blood (plasma and serum) of healthy volunteers and people suffering from CHF was used in the form of Excel tables containing data on the values of surface tension and dilatational viscoelasticity, which characterize viscoelastic properties of surface (interfacial) layers. Baseline data also included the sex and age of the patient. The problem of classifying time series, which arises when analyzing the results of tensiometric analysis, is solved. For the classification of time series, it is proposed to use a neural network. An analysis of neural network methods for classifying time series has been carried out. Using the Keras library, a recurrent neural network was implemented, which ensured a classification accuracy of 99%. The presented approach has demonstrated better performance in terms of accuracy compared to the existing machine learning approaches. The use of the developed neural network model in medical practice will significantly increase the reliability of diagnosing CHF. In addition, the results of the study indicate the possibility of upgrading tensiometers by eliminating the technical elements that provide forced oscillations of the drop, which will significantly reduce the cost of the device and reduce the time of the experiment.