
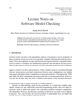
Deep manifold learning has achieved significant success in handling visual tasks by using Symmetric Positive Definite (SPD) matrices, particularly within multi-scale submanifold networks (MSNet). This network is capable of extracting a series of main diagonal submatrices from SPD matrices. However, these submanifolds do not take into account the distribution of the submanifolds themselves. To address this limitation and introduce batch normalization tailored to submanifolds, we devise a submanifold-specific normalization approach that incorporates submanifold distribution information. Additionally, for submanifolds mapped into Euclidean space, considering the weight relationships between different submanifolds, we propose an attention mechanism tailored for log mapped submanifolds, termed submanifold attention. Submanifold attention is decomposed into multiple 1D feature encodings. This approach enables the capture of dependencies between different submanifolds, thus promoting a more comprehensive understanding of the data structure. To demonstrate the effectiveness of this method, we conducted experiments on various visual databases. Our results indicate that this approach outperforms the MSNet.