
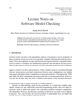
The important process of time series analysis for public health data is to determine target data as a semantic discrete value, according to a context from continuous phenomenon around our circumstance. Typically, each field of experts has their own fields’ specific and practical knowledge to specify an appropriate target part of data which contains the key features of their intended context in each analysis. Those are often implicit, thus not defined as systematically and quantitatively. In this paper, we present a context-based time series analysis and prediction method for public health data. The most essential point of our approach is to express a basis of time series context as the combination of the following 5 elements (1: granularity setting on time axis, 2: feature extraction method, 3: time-window setting, 4: differential computing function, and 5: pivot setting) to determine target data as semantic discrete values, according to the time series context of analysis for public health data. One of the main features of our method is to create different results by switching time series contexts. The method realizes 1) introducing a new normalization (context expression) method to fix a target reference data for time series analysis and prediction according to a context, and 2) presenting a process to generate semantic discrete values reflecting the 5 elements. And the significant features of the proposing method are 1) our context definition realizes the closed world of the semantic differential computing on time axis from the viewpoint of database system, and 2) the 5 elements enable to explicit and quantify experts’ semantic viewpoint of specifying a certain reference data according to a context for each analysis and prediction. As our experiment, we have realized analysis and prediction by applying actual public health data. The results of the experiments show the prediction feasibility of our method in the field of public health data, effectiveness to generate results for discussion regarding switching context, and applicability to express time series context of an expert knowledge for analysis and prediction as combination of the 5 elements to make the knowledge explicit and quantitative expression.