
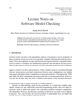
Chronic illnesses like cancer and diabetes are a big deal worldwide, especially in poorer countries. They’re tough on healthcare systems and people’s wallets. Various strategies, like early detection and better management, can help. Machine learning (ML) is getting really good at helping us understand and manage these diseases. Within ML, something called classification algorithms are super useful in figuring out who’s at higher risk for these diseases by sifting through things like medical records and lab results. One standout technique within these algorithms is neural networks, particularly a type called ‘shallow neural networks’ or RBF-NNs. In this investigation, a tweaked version of RBF-NNs namely ‘Radius Local k-Point Radial Basis Function (RLRBF) Neural Networks’ is paid attention to, to see how good they are at classifying people based on their risk of getting a chronic illness. Generally, the two objectives are firstly, to test out how effective this specific type of neural network is, and secondly, to provide useful insights into identifying chronic diseases. Our findings could be a big help for doctors and patients in the future.