
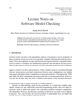
The aim of global distribution network optimization is to optimize the flow of goods between logistics nodes, leading to more efficient and compact packing. As a result, this optimization helps to reduce the shipping cost, which is calculated based on the weight and volume of package after cartonization. Shipping cost is calculated from chargeable weight. In this optimization problem, the routing or rerouting of products or raw materials would result in a new shipment network. To compare the performance of various shipment networks, we use the logistics cost of all shipments within a past time window as the evaluation criteria. Hence, when dealing with the routing/rerouting of numerous types of products/raw materials and having to consider numerous central distribution centers (CDC), a multitude of shipment network configurations would arise. The logistics cost for each routing/rerouting affects other networks and in turn, requires the logistics cost of all other shipment networks to be recomputed as well. Given the enormity of shipments in each network, it is infeasible to employ a cartonization solver to pack and then compute the chargeable weight of the shipments. Chargeable weight is the greater of actual weight and the volumetric weight of the carton after packing. In this paper, a neural network model is applied to predict the chargeable weight of shipments. Conventional machine learning models, such as random forest and support vector regression are used as the benchmark models. Moreover, to further reduce the overall mean error ratio, we propose using exact algorithm and Red Jasper’s cartonization solver to calculate the chargeable weight for small shipments as this combined method runs fast and results in minimal error. As for large complex shipments, we propose using machine learning method to approximate the chargeable weight. Based on real data provided by one of the top five semiconductor equipment makers in the world for experimentation, results suggest that our method achieves a significant improvement in computational speed while maintaining a low mean error.