
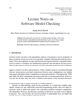
The appearance of new trends in the field of cognitive neuroscience, for example object persistence, has paved the way for the evolution of deep CNNs into Siamese Neural Network architectures such as OPNet. These networks allow for image recognition without the need for expensive labelled data. In this work, we apply this technology to a small Spanish tech e-commerce struggling with the production of their customizable products. Our goal was to automatically identify each product’s order in the company’s internal system by matching photos of the products taken by workers with system-generated images. After testing various architectures, we achieved 91% accuracy with a triplet loss model using deep CNN embedding networks. The algorithm was trained on a dataset of 9696 unique product images captured in the company’s production department. The paper details the technical aspects of the Siamese Neural Network architecture, including the triplet loss and SoftMax distance function used to train it. Our results demonstrate the potential of these deep learning models to generate practical benefits for firms, since it reduces human errors, while improving the effectiveness and efficiency of the company’s internal processes.