
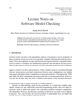
Distributed Space Systems (DSS) are gaining prominence in the space industry due to their ability to increase mission performance by allowing cooperation and resource sharing between multiple satellites. In DSS where communication between heterogeneous satellites is necessary, achieving autonomous cooperation while minimizing energy consumption is a critical requirement, particularly in sparse constellations with nano-satellites. In order to minimize the functioning time and energy consumed by the Inter-Satellite Links (ISL) established for satellite-to-satellite communication, their temporal encounters must be anticipated. This work proposes an autonomous solution based on Supervised Learning that allows heterogeneous satellites in circular polar Low-Earth Orbits to predict their encounters, given the Orbital Elements (OE) and assuming isotropic antenna patterns. The model performance is evaluated and compared in two different scenarios: 1) a simplified scenario assuming that satellites follow Kepler orbits and 2) a realistic scenario assuming that satellites follow Simplified General Perturbations 4 (SGP4) orbits. This work could be considered the first stage of a promising and alternative approach in the field of DSS.