
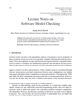
In recent years, traffic conditions in centralised cities have become more severe. To optimise public resources and reduce congestion, transportation departments rely on traffic prediction. However, unexpected events, for instance, rainfall can impact traffic conditions, which necessitates the introduction of weather elements to improve prediction results. Moreover, most of the existing works characterise the relationship between weather and traffic by simply combining these two issues together. Without carefully designing a structure that captures the inter-dependency between weather and traffic data, it is impossible to produce accurate predictions within a reasonable computational time. To address this issue, we propose a Weather-Aware Graph Attention Network (WeaGAN) that adapts an encoder-decoder architecture with weather attention mechanisms and a gate to model the complex spatial-temporal inter-dependency between weather and traffic adaptively. We further design a self-attention mechanism to improve prediction accuracy. Our experiments on a standard real-world dataset show that, compared to the state-of-the-art, WeaGAN: (i) can improve the prediction accuracy by up to 29%; and (ii) is efficient in terms of saving up to 61% of the computation time.