
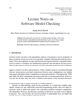
Hierarchical Text Classification (HTC) has recently gained traction given the ability to handle complex label hierarchy. This has found applications in domains like E- commerce, customer care and medicine industry among other real-world applications. Existing HTC models either encode label hierarchy separately and mix it with text encoding or guide the label hierarchy structure in the text encoder. Both approaches capture different characteristics of label hierarchy and are complementary to each other. In this paper, we propose a Hierarchical Text Classification using Contrastive Learning Informed Path guided hierarchy (HTC-CLIP), which learns hierarchy-aware text representation and text informed path guided hierarchy representation using contrastive learning. During the training of HTC-CLIP, we learn two different sets of class probabilities distributions and during inference, we use the pooled output of both probabilities for each class to get the best of both representations. Our results show that the two previous approaches can be effectively combined into one architecture to achieve improved performance. Tests on two public benchmark datasets showed an improvement of 0.99 – 2.37% in Macro F1 score using HTC-CLIP over the existing state-of-the-art models.