
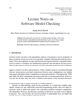
Ontologies represent human domain expertise symbolically in a way that is accessible to human experts and suitable for a variety of applications. As a result, they are widely used in scientific research. A challenge for the neuro-symbolic field is how to use the knowledge encoded in ontologies together with sub-symbolic learning approaches. In this chapter we describe a general neuro-symbolic architecture for using knowledge from ontologies to improve the generalisability and accuracy of predictions of a deep neural network applied to chemical data. The architecture consists of a multi-layer network with a multi-step training process: first, the network is given a self-supervised pre-training step with a masked language step in order to learn the input representation. Second, the network is given an ontology pre-training step in which the network learns to predict membership in the classes of the ontology as a way to learn organising knowledge from the ontology. Finally, we show that visualisation of the attention weights of the ontology-trained network allows some form of interpretability of network predictions. In general, we propose a three-layered architecture for neuro-symbolic integration, with layers for 1) encoding, 2) ontological classification, and 3) ontology-driven logical loss.