
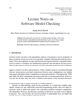
The prediction made by a learned model is rarely the end outcome of interest to a given agent. In most real-life scenarios, a certain policy is applied on the model’s prediction and on some relevant context to reach a decision. It is the (possibly temporally distant) effects of this decision that bring value to the agent. Moreover, it is those effects, and not the model’s prediction, that need to be evaluated as far as the agent’s satisfaction is concerned. The formalization of such scenarios naturally raises certain questions: How should a learned model be integrated with a policy to reach decisions? How should the learned model be trained and evaluated in the presence of such a policy? How is the training affected in terms of the type of access that one has on the policy? How can the policy be represented and updated in a way that is cognitively compatible with a human, so that it offers an explainable layer of reasoning on top of the learned model?
This chapter offers a high-level overview of past work on the integration of modular reasoning with autodidactic learning and with user-driven coaching, as it applies on neural-symbolic architectures that combine sequentially a neural module with an arbitrary symbolically represented (and possibly non-differentiable) policy. In this context, the chapter offers responses to the questions above when the policy can be reasoned with only in a deductive manner, or in a deductive and an abductive manner. It further discusses how the policy can be learned / updated in an elaboration-tolerant and cognitively-light manner through machine coaching, and highlights the connections of the dialectical coaching process with the central role that argumentation plays in human reasoning.