
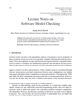
Radiography is used for initial diagnosis and postoperative follow-up. If a radiograph is deemed unsuitable for diagnosis, it is rejected. Retaking a radiograph is disadvantageous for the patient because it prolongs the examination time and increases the radiation dose. Skull radiography is the position in which retaking occurs most frequently. In skull radiography, the patient’s rotational direction is estimated from minute changes in the inner ear’s structure in the lateral skull radiograph. When retaking, the amount of correction for patient positioning is generally estimated from the errors in the rejected image through empirical evidence. Therefore, considerable expertise is needed to correct the positioning appropriately, and inexperienced radiologic technologists take considerable time to estimate this error. This study aimed to estimate the patient’s angle from lateral skull radiographs to compensate for radiologic technologists’ lack of experience and reduce the burden on patients. The radiograph is a simulated 2-D line-integral projection of a 3-D CT image, and we developed an estimation method using deep learning with supervised training. The network is based on a re-scaled ResNet. The patient’s angle was estimated in the lateral and superior-inferior directions. We evaluated the accuracy of estimation with the projected images of 256 simulated cases. The estimation errors were 0.48 ± 0.41° and 0.55 ± 0.50° in the lateral and superior-inferior angles, respectively. These findings suggest that a patient’s angle can be accurately estimated from a radiograph using deep learning, compensating for the lack of experience and reducing retaking time.