
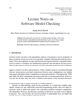
In order to improve the overall economy of fuel cell vehicle, this paper proposes an energy management strategy based on the Car-Following Model of Long Short-Term Memory Neural Network (LSTM-CFM), which classified vehicles according to the Vehicle_ID, Frame_ID, Global_Time and other core data based on the vehicle information collected from the Next Generation Simulation (NGSIM) data set on the US 101 highway in south California. The two adjacent vehicles were identified and the data were extracted and processed into the LSTM-CFM for training. In the simulation stage, UDDS (a standard driving condition) is taken as the driving condition of the followed vehicle and 50m is taken as the initial distance between the following vehicle and the target vehicle. Under these initial conditions, we can get the speed prediction data of target vehicle based on the Car-Following model. The energy management strategy is designed based on the principle of minimum equivalent fuel consumption, and the equivalent factor can be solved by using the speed prediction. The simulation results show that the economy of the fuel cell vehicle under the LSTM-CFM energy management strategy is better than that of the rule-based strategy, which verifies the effectiveness of the LSTM-CFM energy management strategy.