
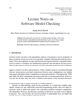
Aiming at the problem that the staff do not wear safety helmets or even wear safety helmets in the construction and maintenance of new energy charging stations, an improved YOLOv3 safety helmet detection method is designed in this paper. Firstly, the 128*128 feature map output is added based on the three feature map output of YOLOv3 algorithm, and then the FI module is added to fuse the four scale feature information to improve the detection accuracy of small targets. Firstly, on the basis of the 3 feature map outputs of the YOLOv3 algorithm, 128*128 feature map outputs are added, and then the FI module is added to fuse the information of the 4 scale feature information to improve the detection accuracy of small targets; Secondly, the DIoU function is used to optimize the boundary frame loss function, so that the boundary frame regression is more accurate. Compared with the original algorithm YOLOv3, the proposed algorithm has higher detection accuracy, shorter time consuming and meets the requirements of real-time detection. Compared with other advanced algorithms, the robustness, detection accuracy and detection speed of the proposed algorithm are better, which can provide technical reference for avoiding staff safety hazards.