
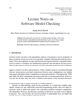
The current approaches for diagnosing mental disorders rely heavily on self-reported and clinical interview ratings. The development of an automatic recognition system assists in the early detection and discovery of biological markers for diagnostic purposes. In this paper, develops a multimodal machine learning model, where it processes multiple modalities like visual, acoustic and textual features using a cross-modality correlation. The study uses a Denoising Autoencoder that finds the multimodal representations and then it adopts Fisher Vector encoding to form session-level descriptors. Paragraph Vector (PV) are used for textual modality that embeds the interview sessions transcripts into document representations that tends to capture the mental disorder cues. Finally, the textual and audio-visual features are fused before training the 3-layered denoising autoencoder (DAE) with Residual Neural Network classifier. The proposed model is validated using two bipolar disorders that includes depression and bipolar disorder. The study uses two different datasets including Extended Distress Analysis Interview Corpus (E-DAIC) and Bipolar Disorder Corpus (BDC) to analyse the depression and bipolar disorders. The experimental evaluation is conducted to show the performance improvement using the proposed multimodal model than other state-of-the art methods in detecting the depression and bipolar disorders. The results of simulation show that the proposed method obtains an improved detection rate than existing models.