
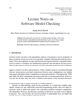
The new forms of networks labeled IoT are relatively new and which become buzz in this decade. The network architecture lets any smart device loosely connect to the Internet under internet protocol. However, the other dimension of this network facilitates intruders to access the network with no critical efforts. The context of intrusions has been delineated as intrusion practices of other devices connected to an IoT network that are connected to external networks through a gateway. Vice versa, the compromised IoT network intends to communicate with external devices or networks to perform intrusion practices. In this regard, intrusion detection through machine learning demands significant feature selection and optimization techniques. This manuscript endeavored to demonstrate the scope distribution diversity assessment methods of traditional statistical practices toward feature selection and optimization in this regard, the contribution “Distribution Diversity Method of Feature Optimization (DDMFO) to Protect Intrusion Practices on IoT Networks” of this paper uses the Dice Similarity Coefficient procedure to pick the optimum characteristics for the training of the classifier. The classifier that has been adopted in this contribution is Naïve Bayes, trained by the features selected by the proposal. The experimental research concludes the significance of the taxonomy, which demonstrates substantial accuracy and minimal false alarm.