
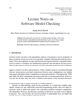
Nowadays, solar spectral irradiances are modelled by solar activity indices, which are used to identify the solar energy absorbed in the environment. This paper devises the Deep LSTM model for predicting solar activity using Sunspot Number (SSN) and Solar Radio Flux (SRF). The processing steps involved in the solar activity prediction are technical indicator extraction and solar activity prediction. In this paper, the solar indices are considered as an input of solar activity prediction, which is acquired from the solar cycle progression dataset. The technical indicators, like Simple Moving Average (SMA), Average True Range (ATR), Relative Strength Index (RSI), William’s %R, Stochastic %D, and Commodity Channel Index (CCI)are extracted for attaining a better prediction performance. In addition, the solar activity prediction is carried out using Deep LSTM based on SSN and SRF. The Deep LSTM is an effective deep learning technique that is widely utilized for prediction purposes since it has a better prediction ability. Moreover, the experimental result demonstrates that the devised Deep LSTM attained the minimum Mean Absolute Error (MAE), Mean Squared Error (MSE) and Root Mean Squared Error (RMSE) of 1.186, 2.869 and 1.693, correspondingly.