
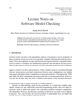
The shifting of the neutral axis in the cross section of ship structures is an important result of progressive collapse analyses. The main purpose of the present study is to apply a Deep Neural Network (DNN) method to linear systems and estimate in a relatively short time span the shift of the neutral axis for intact and damaged ships. First, the initial source data related to the intact condition and to several symmetric damaged grounding scenarios of five different vessels (Double Hull Oil Tanker, Single Hull Tanker, 1350TEU Container Vessel, 3500TEU Container Vessel, Bulk Carrier) have been determined with a self-developed code based on the well-established Smith method. With the application of the DNN, the shift of the neutral axis has been predicted for a set of completely new damage scenarios of a ship cross section, demonstrating that the deep neural network approach can estimate the neutral axis performance. The successful prediction obtained within this paper will lead to the DNN’s application for computing the ultimate strength capacity.