
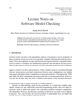
Data quality in health research encompasses a broad range of aspects and indicators. While some indicators are generic and can be calculated without domain knowledge, others require information about a specific data element. Even more complex are indicators addressing contradictions, that stem from implausible combinations of multiple data elements. In this paper, we investigate how contradictions within interdependent categorical data can be identified and if they give additional information about possible quality issues, their cause, and mitigation options. The 19 data elements that represent four biosample types including their pre-analytic states within the DZHK Biobanking basic set are exported to the CDISC Operational Data Model (ODM), transformed and loaded into a tranSMART instance. Through the implementation of a data quality assessment workflow as a SmartR plug-in, statistical information about the domain-specific consistency of interdependent values are retrieved, assessed, and visualized. Data quality indicators have been selected for the assessment according to common recommendations found in the literature. Different contradictions could be discovered in the dataset including mismatch of interdependent values in the pre-analytic states of blood and urine samples, as well as primary and aliquoted samples. The overall assessment rating shows that 99.61% of the interdependent values are free of contradictions. However, measures within the EDC design to avoid contradictions may result in overestimated missing rates in automatic, item-based quality assessment checks. Through consistency checks on interdependent categorical features, we demonstrated that consistency flaws can be found in the categorical data of biobanking metadata and that they can help to detect issues in the data entry process. Our approach underscores the importance of domain knowledge in the definition of the consistency rules but also knowledge about the EDC implementation of such consistency rules to consider the impact on item-based quality indicators.