
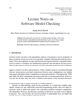
Categories are important elements of databases of Product Listings, for e-commerce platforms, or of Points of Interest (POIs), for location-based services. However, category annotations are often incomplete, which calls for automatic completion. Hierarchical classification has been proposed as a solution to impute missing annotations. We address this task in one of Naver’s production databases (POIs), in order to enhance its quality. In real-life applications, like ours, however, it is unrealistic to count on the existence of a perfectly annotated training set, and noisy training labels prevent us from casting the task as a straightforward classification problem. In order to overcome this difficulty, we propose an approach that takes into account the type of noise in the training set. We identified that the main deficiency is that the training labels tend to be under-specified i.e. they point to categories found at higher levels of the hierarchy than the correct ones. This results in a lot of under-represented and a few over-represented categories. We call categories that are over-represented, due to under-specified labels, joker classes. To allow robust learning in the presence of joker classes we propose a simple and effective approach: First, we detect problematic categories, i.e. joker classes, based on the misclassifications of an initial hierarchical classifier. Then we re-train from scratch, introducing a weight to the standard cross-entropy loss function that targets incorrect predictions related to joker classes. Our model has enabled the correction of thousands of POIs in our production database.