
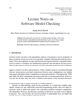
Social robotics is an emerging area that foster the integration of robots and humans in the same environment. With this objective, robots include capacities such as the detection of emotions in people to be able to plan their trajectory, modify their behavior, and generate a positive interaction with people based on the information analyzed. Several algorithms developed for robots to accomplish different tasks, such as people recognition, tracking, emotion detection, demonstrate empathy, need large and reliable datasets to evaluate their effectiveness and efficiency. Most existing datasets do not consider the first-person perspective from the sensory capacity of robots, but third-person perspective from out of the robot cameras. In this context, we propose an approach to create datasets with a robot-centric perspective. Based on the proposed approach, we made up a dataset with 23,222 images and 24 videos, recorded from the sensory capacity of a Pepper robot in simulated environments. This dataset is used to recognize individual and group emotions. We develop two virtual environments (a cafeteria and a museum), where there are people alone and in groups, expressing different emotions, who are then captured from the point of view of the Pepper robot. We labeled the database using the Viola-Jones algorithm for face detection, classifying individual emotions into six types: happy, neutral, sad, disgust, fear, and anger. Based on the group emotions observed by the robot, the videos were classified into three emotions: positive, negative, and neutral. To show the suitability and utility of the dataset, we train and evaluate the VGG-Face network. The efficiency achieved by this algorithm was 99% in the recognition of individual emotions and the detection of group emotions is 90.84% and 89.78% in the cafeteria and museum scenarios, respectively.