
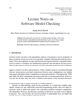
Filters pruning methods are widely used to accelerate the inference process of Deep Neural Networks (DNNs). Among them, Soft Filter Pruning (SFP) has achieved increasing attention due to its compatibility and flexibility. However, conventional SFP directly sets the pruned filters to zero during training phase, discarding the training information of the pruned filter completely. In order to solve the above problem, this paper proposed a soft and smooth pruning method to retain the training information among pruned filters. Instead of the zeroing the pruned filters, our method imposes a filter weakening strategy, which gradually forces the pruned filters to zeros. Such a gradual pruning framework will give the pruned model more chances to recover the lost information and boost the pruning performance. To verify the the actual effectiveness of our proposed method, we conduct several experimental results on one dataset of metal surface images captured in a controlled industrial environment, i.e.,KSDD, using Resnet-20 with various pruning rates. Experimental results show that our filter weakening strategy consistently achieves superior performance over the compared methods, especially when a large amount of filters is pruned. By pruning 60% of filters, our method only drops 2.52% on Accuracy.