
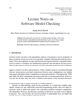
The early detection and treatment of neoplasms, and in particular the malignant, can save lives. However, identifying those most at risk of developing neoplasms remains challenging. Electronic Health Records (EHR) provide a rich source of “big” data on large numbers of patients. We hypothesised that in the period preceding a definitive diagnosis, there exists a series of ordered healthcare events captured within EHR data that characterise the onset and progression of neoplasms that can be exploited to predict future neoplasms occurrence. Using data from the EHR of the Ministry of National Guard Health Affairs (MNG-HA), a large healthcare provider in Saudi Arabia, we aimed to discover health event patterns present in EHR data that predict the development of neoplasms in the year prior to diagnosis. After data cleaning, pre-processing, and applying the inclusion and exclusion criteria, 5,466 patients were available for model construction: 1,715 cases and 3,751 controls. Two predictive models were developed (using Decision tree (DT), and Random Forests (RF)). Age, gender, ethnicity, and ICD-10-chapter (broad disease classification) codes as predictor variables and the presence or absence of neoplasms as the output variable. The common factors associated with a diagnosis of neoplasms within one or more years after their occurrence across all the models were: (1) age at neoplasms/event diagnosis; (2) gender; and patient medical history of (3) diseases of the blood and blood-forming organs and certain disorders involving immune mechanisms, and (4) diseases of the genitourinary system. Model performance assessment showed that RF has higher Area Under the Curve (AUC)=0.76 whereas the DT was less complex. This study is a demonstration that EHR data can be used to predict future neoplasm occurrence.