
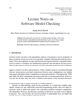
The ongoing COVID-19 pandemic brings new challenges and risks in various areas of our lives. The lack of viable treatments is one of the issues in coping with the pandemic. Developing a new drug usually takes 10-15 years, which is an issue since treatments for COVID-19 are required now. As an alternative to developing new drugs, the repurposing of existing drugs has been proposed. One of the scientific methods that can be used for drug repurposing is literature-based discovery (LBD). LBD uncovers hidden knowledge in the scientific literature and has already successfully been used for drug repurposing in the past. We provide an overview of existing LBD methods that can be utilized to search for new COVID-19 treatments. Furthermore, we compare the three LBD systems Arrowsmith, BITOLA, and SemBT, concerning their suitability for this task. Our research shows that semantic models appear to be the most suitable for drug repurposing. Nevertheless, Arrowsmith currently yields the best results, despite using a co-occurrence model instead of a semantic model. However, it achieves the good results because BITOLA and SemBT currently do not allow for COVID-19 related searches. Once this limitation is removed, SemBT, which uses a semantic model, will be the better choice for the task.