
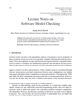
It is highlighted for machine learning models implementing functions based on data training without program coding. Artificial neural network is one of the efficient machine learning models. Different from the other machine learning models like artificial neural network, we have presented semantic computing models which represent “meaning” of machine learning results. In our model, semantic spaces are created based on training data sets. Data calculations are performed on the space. Data are mapped to semantic spaces and presented as points in semantic spaces. The mapped positions of data represent the “meaning” of data. In this paper, we first present our new discovery in the formation of semantic spaces. We use the word “matter” to represent features of semantic spaces which are related to the non-temporal data. As the same time, we use the word “dark-matter” to represent the features of semantic spaces which are temporally changed. We use the word “energy” to represent matrixes which are used in the semantic computations to generate output data. We reveal that the “dark-matter” is the spatiotemporal matrix and present a mechanism of “memory” for implementing the semantic computation. The most important contribution of this paper is that we developed a new mechanism for implementing machine learning with “knowledge” in the “memory.” In the paper, we use case studies to illustrate the concepts and the mechanism. At the beginning, we present an example on creating a semantic space from a “chaotic state” to an “ordered state.” After that, we use examples to illustrate the mechanism of the “memory” and the semantic computation. The space expansion and the space division are also illustrated by examples.