
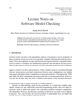
Human-robot interactive decision-making is increasingly becoming ubiquitous, and explainability is an influential factor in determining the reliance on autonomy. However, it is not reasonable to trust systems beyond our comprehension, and typical machine learning and data-driven decision-making are black-box paradigms that impede explainability. Therefore, it is critical to establish computational efficient decision-making mechanisms enhanced by explainability-aware strategies. To this end, we propose the Trustworthy Decision-Making (TDM), which is an explainable neuro-symbolic approach by integrating symbolic planning into hierarchical reinforcement learning. The framework of TDM enables the subtask-level explainability from the causal relational and understandable subtasks. Besides, TDM also demonstrates the advantage of the integration between symbolic planning and reinforcement learning, reaping the benefits of both worlds. Experimental results validate the effectiveness of proposed method while improving the explainability in the process of decision-making.