
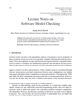
Corporate bond default risk prediction is important for regulators, issuers and investors in the bond market. We propose a new approach for multi-class imbalanced corporate bond risk prediction based on the OVO-SMOTE-Adaboost ensemble model, which integrates the one-versus one (OVO) decomposition method, the synthetic minority over-sampling technique (SMOTE) and the Adaboost ensemble method. We categorize corporate bond default risk into three classes: very low default risk, relatively low default risk and high default risk, which is more scientific than the traditional two-class bond default risk, and carry out empirical experiments by respectively using DT, SVM, Logit and MDA as basic classifiers. Empirical results show that the prediction performance of the OVO-SMOTE-Adaboost (DT) model is overall better than the other three ensemble models such as OVO-SMOTE-Adaboost (SVM), OVO-SMOTE-Adaboost (Logit) and OVO-SMOTE-Adaboost (MDA). In addition, the OVO-SMOTE-Adaboost (DT) model greatly outperforms the OVO-SMOTE (DT) model, which is a single classifier model based on OVO and SMOTE without Adaboost. Therefore, the OVO-SMOTE-Adaboost (DT) model has satisfying performance of multi-class imbalanced corporate bond default risk prediction and is of great practical significance.