
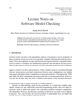
A sampling method is one of the popular methods to deal with an imbalance problem appearing in machine learning. A dataset having an imbalance problem contains a noticeably different number of instances belonging to different classes. Three sampling techniques are used to solve this problem by balancing class distributions. The first one is an undersampling technique removing noises from a class having a large number of instances, called a majority class. The second one is an over-sampling technique synthesizing instances from a class having a small number of instances, called a minority class, and the third one is the combined technique of both undersampling and oversampling. This research applies the combined technique of both undersampling and oversampling via the mass ratio variance scores of instances from each individual class. For the majority class, instances with high mass ratio variances are removed whereas for the minority class, instances with high mass ratio variances are used in synthesizing minority instances. The results of this proposed sampling technique help improve recall over standard classifiers: a decision tree, a random forest, Linear SVM, MLP on all synthesized datasets; however it may have low precision. So the combined measure of precision and recall is used, F1-score. Recall and F1-scores of synthesized datasets and UCI datasets are significantly better for collections of datasets having small imbalance ratio. Moreover, the Wilcoxon signed-rank test is used to confirm the improvement for datasets having imbalance ratio smaller than or equal to 0.2.