
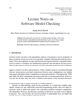
With the widely use of recommendation systems in various mobile applications, privacy leakage has been a longstanding threat, for which many researchers have come up with a great number of methods that achieve the protective effect to a certain extent. However, the protection scope of these methods is limited, especially in the protection of original data. To address this issue, we propose a data perturbation based Rényi differential privacy algorithm to protect the SVD recommendation model. This paper uses the data perturbation method to perturb the original training dataset in the data preprocessing stage, then leverages the perturbed data to train the SVD model, and the unperturbed data is used as a test set to verify the accuracy of the model. Compared with the objective perturbation, gradient perturbation, and output perturbation, the data perturbation can protect a broader range and realize the corresponding functions of the other three perturbed methods by using the post-processing property of differential privacy. Experimental results show that the proposed method can effectively protect user privacy, improve the effectiveness of data, and generate better recommendation results without seriously affecting the accuracy of the model.