
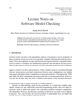
Segmentation of Text, the undertaking of partitioning a record obsessed by adjoining sections dependent on its semantic design, is a longstanding test in language understanding. Each segment has its applicable significance. Those segments arranged as phrase, text group, point, express or any data unit relying upon the errand of the content examination. This paper proposes the profound learning-based content segmentation strategies in NLP where the content has been portioned utilizing quick tangled neural organization. We propose a bidirectional LSTM prototype where text group embedding is gotten the hang of utilizing fast RNNs and the phrases are fragmented dependent on context-oriented data. This prototype can consequently deal with variable measured setting data and present an enormous new dataset for text segmentation that is naturally divided. Besides, we build up a segmentation prototype dependent on this dataset and show that it sums up well to inconspicuous regular content. We find that albeit the segmentation precision of FRNN with Bi-LSTM segmentation is advanced than some other segmentation techniques. In the proposed framework, every content is resized obsessed by required size, which is straightforwardly exposed to preparation. That is, each resized text has foreordained and these phrases are taken as fragmented content for preparing the neural organization. The outcomes show that the proposed framework yields great segmentation rates which are practically identical to that of segmentation-based plans for manually written content.